
International Conference on Statistics and Related Fields (ICON STARF) in honour of Vladimir Koltchinskii
The International Conference on Statistics and Related Fields (ICON STARF) will take place from 3 (arrival date) to 7 June (departure date) 2024 at the Centro Residenziale Universitario di Bertinoro
Speakers
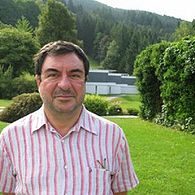
Prof. Lucien Birgé
Université Pierre-et-Marie-Curie