
Programme (7-11 December 2020)
During the winter school, three courses were offered, each consisting of three 90-minute sessions by Cristina Butucea (CREST ENSAE, Université ParisTech), Karim Lounici (CMAP-Ecole Polytechnique) and Stéphane Robin (AgroParisTech/INRA/univ. Paris Saclay & Muséum National d’Histoire Naturelle). In addition, nine doctoral students gave some talks.
Day 1: 7 December
09:00 Welcome and Winter School Introduction
10:00 Non-parametric Inference under Local Differential Privacy, Cristina Butucea
11:30 Discussion break
12:15 Lunch break
14:00 Automatic design of neural networks with Gaussian Processes, Ayman Makki
14:30 Reinforcement Learning Enhanced Heterogeneous Graph Neural Network, Zhiqiang Zhong
Day 2: 8 December
09:00 Statistical inference of incomplete data models to analyse ecological networks, Stéphane Robin
10:30 Break
11:15 Principal Component Analysis: some recent results and applications, Karim Lounici
12:45 Lunch break
14:00 p-Estimation in Mixture Models, Alexandre Lecestre
14:30 Diffusivity Estimation for Activator-Inhibitor Models – Theory and Application to Intracellular Dynamics of the Actin Cytoskeleton, Gregor Pasemann
15:00 Topological Measures for cancer drug sensitivity prediction and repositioning, Apurva Badkas
Day 3: 9 December
09:00 Non-parametric Inference under Local Differential Privacy, Cristina Butucea
10:30 Break
11:15 Statistical inference of incomplete data models to analyse ecological networks, Stéphane Robin
12:45 Lunch break
14:00 Robust estimation of a regression function in exponential families, Juntong Chen
14:30 Exact variation for stochastic heat equation with piecewise constant coefficients and application to parameter estimation, Eya Zougar
Day 4: 10 December
09:00 Non-parametric Inference under Local Differential Privacy, Cristina Butucea
10:30 Break
11:15 Principal Component Analysis: some recent results and applications, Karim Lounici
12:45 Lunch break
14:00 NAV calculation errors and corrections of investment funds: definition and simulation to measure the statistical impact on the NAV time series, Simon Petitjean
14:30 Concentration inequalities on M-estimators for robust mean estimating, Timothée Mathieu
Day 5: 11 December
09:00 Statistical inference of incomplete data models to analyse ecological networks, Stéphane Robin
10:30 Break
11:15 Principal Component Analysis: some recent results and applications, Karim Lounici
12:45 Lunch break
14:00 Special session: EU funding opportunities
About the 3 main courses
Non-parametric Inference under Local Differential Privacy
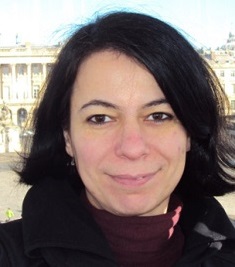
Data privacy protection is a major issue for our society nowadays due to the massive amounts of data collected and stored by many electronic devices at all times, on social networks, in medecine, in finance and so on. This leads to multiple sources of data concerning the same individuals (persons, funds, etc.) that can be easily aggregated in order to identify them. Therefore, privacy preserving mechanisms have to be applied to the data before their public release which implies to quantify the amount of privacy, but also to decide a priori whether collaboration between data holders is possible/authorized or unadvisable/forbidden.
More information: Abstract Non-parametric Inferece under Local Differential Privacy
Principal Component Analysis: some recent results and applications
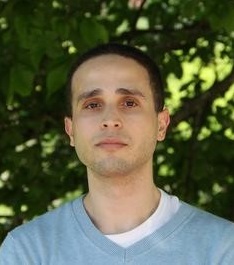
Several recent applications in statistics, machine learning or numerical analysis can be formulated as high-dimensional matrices processing problems. Extracting information efficiently from these objects often require to develop new computationally efficient methods. Understanding how and when these methods work is a fascinating topic of research that require to combine tools from several fields of mathematics: statistics, probability, perturbation theory and convex optimization. In this course, we will review how to use these tools in the context of Principal Component Analysis to analyse the performances of the standard PCA method. Results will include concentration bounds, asymptotic distributions and minimax lower bounds for functionals of spectral projectors. Next, we will explain how to exploit this recent theory to provide some insight into some new or longstanding problems in machine learning including Gaussian mixture, graph clustering, domain adaptation.
Statistical inference of incomplete data models to analyse ecological networks
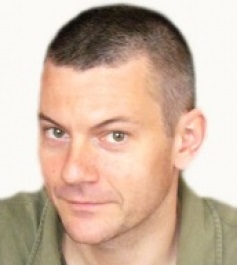
Ecological networks aim at describing the interactions between a set of species sharing a same ecological niche. The interactions constituting a network can be directly observed (e.g. via plant-pollinator contacts) or may need to be reconstructed based on the fluctuations of the species’ abundance across different sites. Statistical models are needed either to describe the organisation (or ‘topology’) of an observed network, or to infer the set of interactions that underlies the joint distribution of the abundances. Various models have been proposed for both purposes.
These lectures will focus on two emblematic families of models. The stochastic block-models (SBM) are dedicated to the topological analysis of observed networks and assumes that species have different roles in the network and that the interaction between them depend on their respective roles. The Poisson log-normal (PLN) model is a joint species distribution model (JSDM) that relies on a Gaussian latent layer. Interestingly, both models are incomplete data models and their statistical inference raise similar issues.
After a brief reminder about most popular methods for the inference of incomplete data models, we will show that they do not apply to SBMs or PLN models. We will introduce inference methods based on variational algorithms, which rely on an approximation of the conditional distribution of the unobserved variables given the observed data. Such algorithms have been shown to be efficient for the inference of a large class of incomplete data models, but their theoretical understanding remains itself incomplete. Eventually, we will discuss various leads to combine variational approximations with statistically grounded estimation procedures.
About the PhD talks
Talk 1: Automatic design of neural networks with Gaussian Processes
Speaker: Ayman Makki
Abstract: Designing neural networks architectures has become a tough challenge since the rise of deep learning. While automatic design of such architectures became a competitive research field, it unveiled several technological bottlenecks of the underlying optimization problem. Among others, it requires to deal with different kinds of variables (continuous, discrete and categorical) while demanding an effective exploration of the research landscape about which we do not have much
prior knowledge. Besides, the high computational cost induced by the training of such networks makes requires optimization methods using only a few cost function evaluation. After having defined the terms of the optimization problem, I will introduce promising research axes to solve those bottlenecks while allowing an improvement of current state-of-the-art methods.
Talk 2: Reinforcement Learning Enhanced Heterogeneous Graph Neural Network
Speaker: Zhiqiang Zhong
Abstract: Heterogeneous Information Networks (HINs), involving a diversity of node types and relation types, are pervasive in many real-world applications. Recently, increasing attention has been paid to heterogeneous graph representation learning (HGRL) which aims to embed rich structural and semantics information in HIN into low-dimensional node representations. To date, most HGRL models rely on manual customisation of meta paths to capture the semantics underlying the given HIN. However, the dependency on the handcrafted meta-paths requires rich domain knowledge which is extremely difficult to obtain for complex and semantic rich HINs. Moreover, strictly defined meta-paths will limit the HGRL’s access to more comprehensive information in HINs. To fully unleash the power of HGRL, we present a Reinforcement Learning enhanced Heterogeneous Graph Neural Network (RL-HGNN), to design different meta-paths for the nodes in a HIN. Specifically, RL-HGNN models the meta-path design process as a Markov Decision Process and uses a policy network to adaptively design a meta-path for each node to learn its effective representations. The policy network is trained with deep reinforcement learning by exploiting the performance of the model on a downstream task. We further propose an extension, RL-HGNN++, to ameliorate the meta-path design procedure and accelerate the training process. Experimental results demonstrate the effectiveness of RL-HGNN, and reveals that it can identify meaningful meta-paths that would have been ignored by human knowledge.
Talk 3: p-estimation in Mixture Models
Speaker: Alexandre Lecestre
Abstract: Finite mixture models are latent variable models used to represent the presence of sub-populations within an overall population, without sub-population identity information. We consider finite
mixture model, where the number of components is known. The problem then consists of estimating the emission distributions (distribution of each sub-population) and the weights (proportion of each sub-
population) of a mixture distribution. For the sake of simplicity, we focus on the specific situation of two-component contamination mixtures with parametric models. After a quick introduction to mixture
models, we try to give some motivation for using rho-estimation. Then we apply rho-estimation in this situation, and try to compare the theoretical guarantees to other methods.
Talk 4: Diffusivity Estimation for Activator-Inhibitor Models – Theory and Application to Intracellular Dynamics of the Actin Cytoskeleton
Speaker: Gregor Pasemann
Abstract: A theory for diffusivity estimation for spatially extended activator-inhibitor dynamics modelling the evolution of intracellular signaling networks is developed in the mathematical framework of stochastic reaction-diffusion systems. In order to account for model uncertainties, we consider the problem of joint estimation of diffusivity and parametrized reaction terms. Our theoretical findings are applied to the
estimation of effective diffusivity of signaling components contributing to intracellular dynamics of the actin cytoskeleton in the model organism Dictyostelium discoideum. This is joint work with Sven Flemming, Sergio Alonso, Carsten Beta, and Wilhelm Stannat.
Talk 5: Topological Measures for cancer drug sensitivity prediction and repositioning
Speaker: Apurva Badska
Abstract: The main challenges in cancer therapy are heterogeneity in patient response, and drug resistance. Cancer heterogeneity and its evolutionary characteristics cause heterogeneity in drug response: not all patients respond to a given drug. For the patients who do respond, resistance to the drug is seen in many cases. Hence, given the time criticality and heavy cost of cancer therapy, one of the aims of ongoing research is prediction of patient-specific drug sensitivity. Also, given the numerous cancer types and their heterogeneous genetic landscape, combined with the long time span and heavy cost of drug development, designing and developing new therapies is prohibitive. Instead, current research focus is on trying to reposition approved drugs for new indications, including cancer. Biological networks are being studied extensively for both these applications, with several different methods of network building and analysis. Several network measures have been proposed over the years, coming from diverse fields such as social networks, psychology, logistics etc. Particularly, biological networks contain encoded information in their structures, and these measures have been used to gain insights into important nodes and node communities and their biologically meaningful interpretations. We examine if topological features of patient-specific cancer networks can be informative features for predicting drug-sensitivity. This investigation also looks into sensitivity predictions for non-cancer drugs, to explore drug repositioning opportunities.
Talk 6: : Robust estimation of a regression function in exponential families
Speaker: Juntong Chen
Abstract: In this talk, we consider the regression framework where the regression function belongs to an exponential family for example logit regression, poisson regression, exponential regression and so on. Our method is based on rho-estimation which is robust. We will show the theoretical performance of our estimator under a suitable parametrisation. And we will introduce various examples to apply our approach.
At the end, we provide a simulation study for calculating our estimator and compare it with maximum likelihood estimator and median-based estimator.
Talk 7: Exact variation for stochastic heat equation with piecewise constant coefficients and application to parameter estimation
Speaker: Eya Zougar
Abstract: We expand the quartic variations in time and the quadratic variations in space of the solution to a stochastic partial differential equations with piecewise constant coefficients, Both expansions allow
us to deduce an estimation method of the parameters appearing in the equation.
Talk 8: NAV calculation errors and corrections of investment funds: definition and simulation to measure the statistical impact on the NAV time series
Speaker: Simon Petitjean
Abstract: Net Asset Value errors are identified as a very critical operational risk for investment funds and particularly mutual funds. Despite mutual fund malfunctions have been intensively studied in the literature, the accounting errors of mutual funds are still to be explored. We provide a detailed definition of the NAV calculation error including a study of the statistical impact on the NAV time series. A unique data
set of NAV errors occurring on Luxembourg mutual funds is combined with the Luxembourg UCITS database in order to bring a new outlook on the phenomenon. We nd that the statistical behavior of the error is not related to the type of error, i.e. pricing, booking, expense error, etc. However, we can distinguish two different statistical behaviors that we can easily relate to operational facts. We simulate the two behaviors, i.e. punctual and gradual errors, with Poisson distribution for the duration of the error and respectively a gamma distribution and a Brownian motion to simulate the impacts of the error on the NAV time series. Our work is particularly interesting for auditors and financial regulators that need accurate tools to control the errors corrected and reported by the management companies.
Talk 9: Concentration inequalities on M-estimators for robust mean estimation
Speaker: Timothée Mathieu
Abstract: In this presentation, we will show how we can study the deviation properties of M-estimators using the tool of influence function. This allows us to control M-estimators (that are defined implicitly) using a sum of i.i.d random variables (which we can easily handle). In a second part, we will use these properties on the problem of robust multivariate mean estimation, we will show some theoretical properties of M-estimators in the multivariate context and we will also illustrate these estimators in simulation.